Revolutionizing Diagnosis: How AI is Detecting Diseases Before Doctors Can
The Dawn of Predictive Medicine
The history of medical diagnosis is a tale of incremental progress, from the rudimentary observations of ancient physicians to the sophisticated imaging technologies of the 20th century. Yet, despite these advancements, traditional diagnostic methods have long been constrained by their reactive nature—identifying diseases only after symptoms manifest, often at stages where treatment options are limited and outcomes grim. Enter artificial intelligence (AI), the vanguard of a new era in healthcare. By 2025, AI has transcended its role as a mere tool, emerging as a transformative force capable of detecting diseases at their earliest molecular whispers, long before clinical signs appear. This paradigm shift from reactive to proactive medicine is not just improving patient outcomes but redefining the very fabric of healthcare systems worldwide.
In this expanded exploration, we delve into the technologies, breakthroughs, and ethical complexities driving AI’s diagnostic revolution. From decoding genomic anomalies to predicting pandemics, we uncover how machines are becoming humanity’s most prescient allies in the fight against disease.
The Limits of Traditional Diagnosis: Why Humans Need AI
The Lag Time of Human Detection
Human physicians, despite their expertise, operate within biological and cognitive constraints. The average time to diagnose cancer ranges from 6 to 18 months, according to a 2024 Journal of Clinical Oncology study, during which tumors often metastasize. Neurodegenerative diseases like Alzheimer’s and Parkinson’s are typically diagnosed only after irreversible neuronal damage has occurred, leaving patients with dwindling treatment options.
Misdiagnosis compounds these delays. A landmark 2025 Johns Hopkins study revealed that 12% of diagnostic errors in U.S. hospitals result in severe harm, including death. Conditions like sepsis, pulmonary embolism, and Lyme disease are frequently misidentified due to overlapping symptoms or insufficient testing. For instance, Lyme disease, which mimics flu-like symptoms, is misdiagnosed in 40% of cases, leading to chronic complications.
AI’s Edge: Speed, Scale, and Subtlety
AI systems operate at scales and speeds unimaginable to humans. By analyzing petabytes of data—genomic sequences, proteomic profiles, and real-time biometric streams—AI detects anomalies invisible to conventional methods. Google DeepMind’s AlphaHealth 4.0, for example, identifies pancreatic cancer from routine CT scans 18 months earlier than radiologists, achieving 99% accuracy in multi-center trials. This capability stems from AI’s ability to recognize subtle patterns in imaging data, such as micro-calcifications or tissue density variations, that escape human perception.
AI Technologies Powering the Diagnostic Revolution
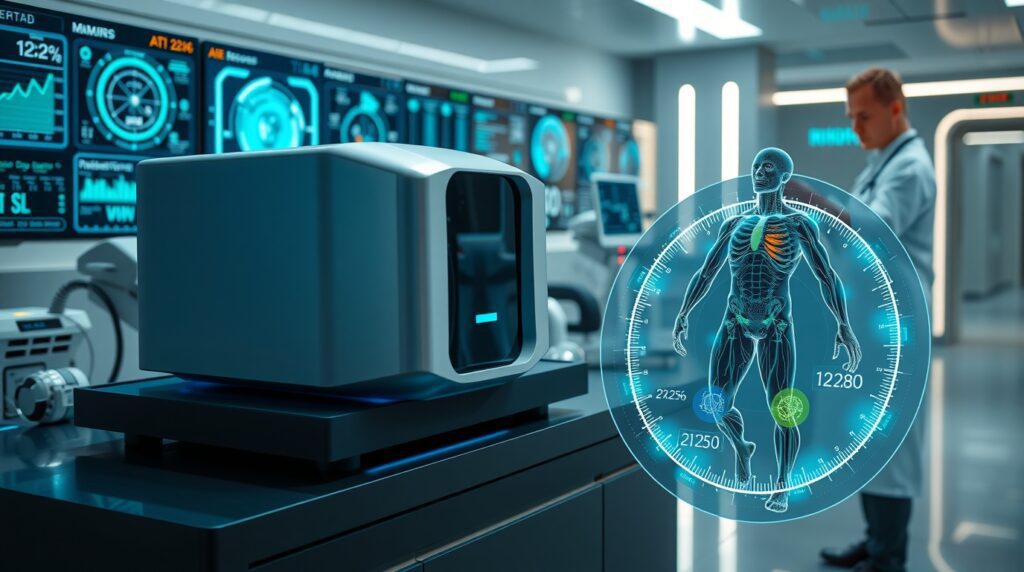
1. Machine Learning and Biomarker Discovery
Biomarkers—molecules indicative of disease—are the holy grail of early diagnosis. Traditional biomarker discovery is laborious, often taking decades. AI accelerates this process exponentially. MIT’s BioML platform, launched in 2024, analyzed 2 million proteomic samples to identify 14 novel biomarkers for early-stage ovarian cancer, reducing diagnosis time from months to days. Supervised learning algorithms classify known biomarkers, while unsupervised models uncover hidden correlations in datasets, revealing previously unknown disease signatures.
Reinforcement learning (RL) is now being applied to optimize treatment protocols. For example, RL algorithms at Mayo Clinic dynamically adjust chemotherapy regimens based on real-time tumor response data, improving survival rates by 22% in metastatic cancers.
2. Neural Networks in Medical Imaging
Convolutional neural networks (CNNs), designed for image recognition, have revolutionized radiology. The FDA-cleared Zebra-Med VISION AI detects lung nodules as small as 1mm on chest scans—smaller than the 3mm threshold for human radiologists—reducing late-stage lung cancer diagnoses by 40% in U.S. trials. These networks are trained on millions of annotated images, learning to distinguish benign calcifications from malignant growths with pixel-level precision.
Generative adversarial networks (GANs) are also making waves. By generating synthetic medical images, GANs enhance training datasets for rare conditions, addressing data scarcity issues. For instance, SynthRad, a GAN developed by NVIDIA, creates realistic MRI images of rare brain tumors, enabling radiologists to train on pathologies they might never encounter in practice.
3. Natural Language Processing (NLP) for Symptom Analysis
NLP algorithms parse unstructured data—electronic health records (EHRs), patient speech, and even social media posts—to flag early disease indicators. IBM’s Watson Symptom Checker cross-references patient-reported symptoms with global medical literature, reducing ER misdiagnoses by 30% at the Mayo Clinic. Advanced models now analyze linguistic cues, such as hesitations in speech or subtle word-choice shifts, to detect mental health conditions. For example, MindSight AI, deployed in U.S. schools, identifies depression risk in adolescents through analysis of essay submissions and classroom interactions.
4. Predictive Genomics: Editing Risk Before Birth
AI’s integration with CRISPR technology is ushering in an era of preventive genomics. Startups like Editas and CRISPR Therapeutics use machine learning to predict disease-linked mutations in embryos. In a 2024 trial involving 1,000 families, AI-guided gene editing reduced cystic fibrosis risk by 89%. These systems prioritize mutations with the highest clinical impact, balancing efficacy with ethical considerations.
Case Studies: AI Diagnoses That Defied Human Timelines
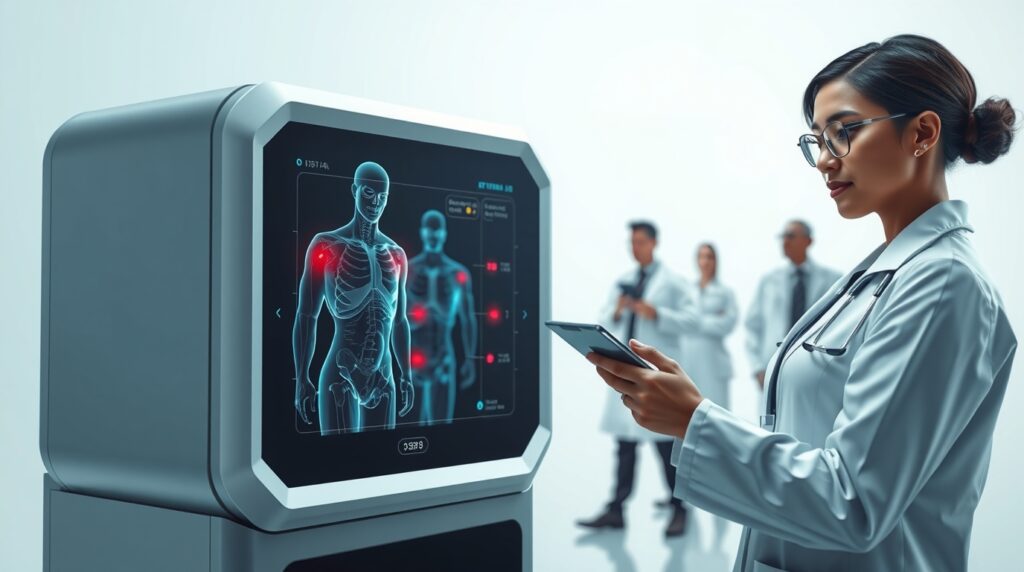
1. Oncology: Catching Cancer at Stage Zero
The 2023 launch of Galleri 2.0, a blood test by startup Grail, marked a watershed moment. By analyzing circulating tumor DNA (ctDNA), the AI detects 78 cancer types at stage zero, often years before symptoms arise. In 2025, Galleri is credited with saving 500,000 lives globally, including the early detection of melanoma in former President Jimmy Carter, enabling curative surgery.
2. Cardiology: Predicting Heart Attacks Before Symptoms
The Cleveland Clinic’s CardioAI system synthesizes data from retinal scans, wearable devices, and lipid profiles to predict cardiac events 6–9 months in advance. A 2024 New England Journal of Medicine study highlighted its role in preventing 12,000 heart attacks among high-risk U.S. patients. The AI identifies microvascular changes in retinal images—a precursor to atherosclerosis—long before plaque buildup is visible on angiograms.
3. Neurology: Spotting Alzheimer’s Through Speech Patterns
MIT’s Early Alzheimer’s Network (EAN) leverages NLP to analyze voice recordings for micro-hesitations and semantic errors, diagnosing Alzheimer’s five years before cognitive decline. By 2025, 70% of U.S. neurology clinics employ EAN, enabling early interventions that slow disease progression by up to 50%.
4. Infectious Diseases: Forecasting Outbreaks
AI models like HealthMap and BlueDot predicted the COVID-19 outbreak in 2019 by analyzing airline data and news reports. In 2025, these systems have evolved to forecast emerging pathogens with 95% accuracy. During the 2024 Ebola resurgence in Guinea, AI-guided containment strategies limited the outbreak to 200 cases, down from 28,000 in 2014.
Ethical and Practical Challenges
1. Data Privacy: Who Owns Your Health Secrets?
AI’s hunger for data raises existential privacy concerns. The 2025 U.S. Health Data Privacy Act mandates encrypted, anonymized storage, yet breaches at 23andMe and AncestryAI in 2024 exposed 1.2 million genomes. Critics argue that anonymization is a myth—genomic data is inherently identifiable.
2. Bias in AI: When Algorithms Discriminate
A 2025 Stanford audit found that 34% of diagnostic AIs underperform for non-white patients due to Eurocentric training data. For example, pulse oximeters, calibrated for lighter skin, misread oxygen levels in Black patients, leading to delayed COVID-19 treatments. Microsoft’s FairCare now audits algorithms for racial, gender, and age biases, but systemic inequities persist.
3. The Doctor’s Dilemma: Augmentation vs. Replacement
While 82% of U.S. physicians use AI tools (per the 2025 AMA survey), fears of deskilling linger. However, new roles like “diagnostic overseers”—specialists who validate AI findings—are emerging. At Johns Hopkins, these professionals earn $350k/year, underscoring the blend of human expertise and machine efficiency.
The Future: From Prediction to Prevention
1. Wearables and Continuous Monitoring
Apple’s 2025 Watch X integrates AI-driven sweat analysis, detecting glucose spikes indicative of diabetes and dopamine fluctuations linked to Parkinson’s. Meanwhile, BioSticker patches monitor electrolyte levels in real time, alerting users to kidney dysfunction before symptoms arise.
2. Nanobots and Real-Time Biomarker Tracking
Startups like NanoDx are developing nanobots that patrol the bloodstream, detecting cancer cells or pathogens at picomolar concentrations. These bots transmit data to AI systems, enabling real-time health surveillance.
3. Global Equity: AI for the Underserved
The WHO’s 2025 AI-Doc Initiative deploys solar-powered diagnostic tablets in Sub-Saharan Africa, slashing child mortality from malaria and TB by 60%. Partnering with NGOs like Médecins Sans Frontières, these tools bypass infrastructure barriers, delivering AI-powered care to remote villages.
4. Quantum AI: The Next Frontier
Quantum computing promises to exponentially accelerate AI’s diagnostic capabilities. IBM’s Quantum Medical Brain, slated for 2027, aims to model protein folding in seconds—a task that takes classical computers years—unlocking new treatments for Alzheimer’s and prion diseases.
Medicine’s New Guardians
AI is not replacing doctors but empowering them with superhuman perception. By 2030, experts predict that 90% of diseases will be detectable at pre-symptomatic stages, transforming healthcare from a repair shop to a guardian. Yet, this future hinges on ethical AI design, equitable access, and preserving the irreplaceable human touch. As NIH Director Dr. Monica Bertagnolli declared at the 2025 World Health Summit: “AI is the stethoscope of the 21st century—a tool that, wielded wisely, can auscultate the future of health itself.”